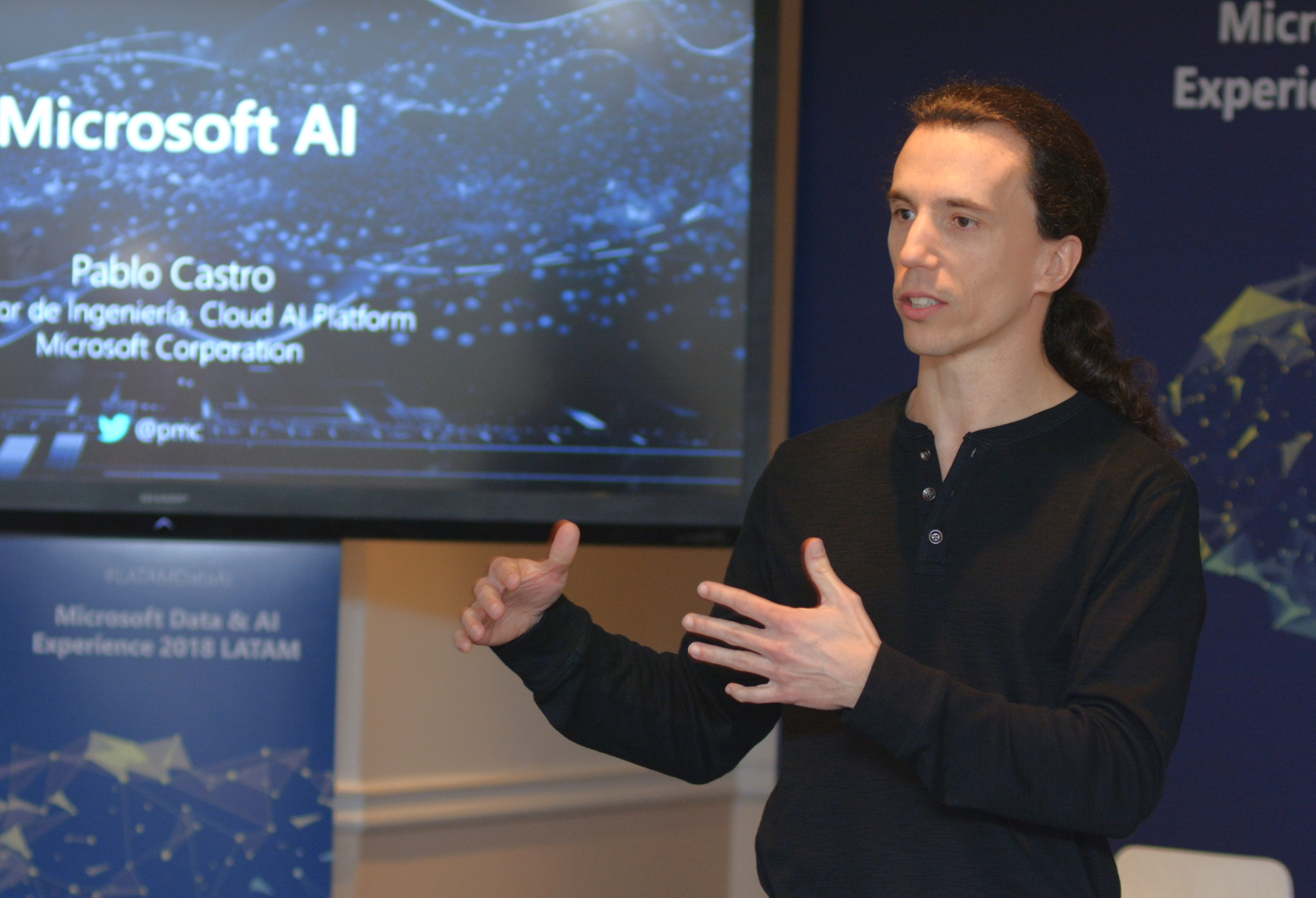
As a distinguished engineer working on the Microsoft Azure AI Search team, Pablo Castro has insight into the inner workings of generative AI, AI search, and the approach that Microsoft is taking to build these systems with its developer ecosystem in mind.
Speaking on a new episode of the Shift AI Podcast, Castro mentions three trends he’s excited about in these areas.
- Models with much longer context length, which allow more data to be taken into consideration when AI responds to each prompt.
- Faster AI models, with speed becoming more important for enabling real-time conversations and decision-making.
- Increasing sophistication of retrieval systems, with different language models and knowledge bases increasingly working together to improve answers.
Castro also hints at upcoming developments from Microsoft.
“We’ve been working hard on the state-of-the-art systems for removing all these concerns around the cost of scale,” he says. “You’ll see us, very soon, talk about a complete shift in the level of scale you can achieve with our retrieval systems for generative AI apps, and a huge improvement in economics.”
One of Castro’s goals, he says, is to ensure that economic concerns don’t limit the amount of data that people apply to artificial intelligence models.
He also cites some specific areas of focus for Microsoft’s Azure AI platform this year:
- Lowering friction: The company is looking to make it easier for customers to create end-to-end AI experiences, including acquiring and preparing data, and ensuring good results.
- Scaling to production: While much of the attention was on proofs of concept in 2023, the focus has expanded this year to help customers integrate AI reliably at scale in secure production environments.
Those are some of the insights from Castro in this episode of Shift AI, a show that explores what it takes to adapt to the changing workplace in the digital age of remote work and AI. We also discuss his early days at Microsoft and explore his role leading the Azure AI team in the discovery of how best to integrate Gen-AI and AI search into business operations.
Listen below, and continue reading for highlights from his comments, edited for context and clarity. Subscribe to the Shift AI Podcast and hear more episodes at ShiftAIPodcast.com.
Role at Microsoft Azure AI: Today I’m spending a lot of time at the intersection of these language models and knowledge. So the question that we tackle with my team is, in a world where you have these language models that are effectively reasoning engines that are very clever, but they don’t know about the stuff that you need them to know about, and we have lots of data, but it’s separated from these models, how do you put them together? That intersection is critical in terms of applying all this fascinating technology to business problems. It’s also, frankly, a lot of fun because it mixes these language modeling problems with knowledge representation problems. How do we integrate these things? How do we take advantage of the strengths of each of these pieces of technology? We’ve been spending a lot of time in this space.
A ‘tight learning loop’: The last 18 months, maybe a little longer, have been a blur. So many of our customers are doing things with Azure OpenAI, and Azure AI Search, and the rest of the Azure AI platform. We’ve learned a ton from people taking this technology and using it for real problems, real business priorities. So that has been fascinating. But also, it’s been a learning opportunity. It’s very humbling when all these people are excited about the thing that you’re offering, and then they go to use it, and they’re like, “Well, we couldn’t because this didn’t work, or this is not what I thought.” So we’re in this very tight learning loop.
The state of AI in 2024: If I look at 2023, I feel it was the year of demos and proofs of concept, where this was so new. All of us wanted to see what it felt like to build one of these things, and everyone took their business problem and gave it a try. It is clear that 2024 is the year all of these things are going to production. So the challenges shift from, “How do I get this off the ground and try one or the other thing?” to “How do I do this securely? How do I know that this integration is going to work well over time?” We’re going to add multiple orders of magnitude in scale. And what does that mean for the systems that are powering these? And do they scale the way you think they will, and would not? I always think about the things we can do for our customers to remove problems for them, so that they can think about the things only they can do because they have the context of their own business.
AI data and security: One of the top questions I hear from customers is, “Well, but no matter what I do with data management, at some point I’m going to take the question and instructions and the grounding information, and I’m going to send it to Azure OpenAI. So you are going to see the data. Are you going to train on that? Are you going to learn from it?” And we made it a point to have a very short and crisp answer, which is, no. We don’t train on customer data. We don’t learn from that data. We don’t use it to improve the models. In fact, we don’t keep it.
The future of work: I’m not a marketing person and I don’t understand many of the choices that we make, but whoever coined Copilot as the thing for Microsoft, they had a very good day. That’s exactly how I think about this current wave of technology. There are these kinds of extensions of your brain that can help you do things that it would not have been practical to do otherwise, while still factoring human ingenuity in the picture.
Listen to the full episode of Shift AI with Microsoft Azure AI Distinguished Engineer Pablo Castro here.